Artificial Intelligence for precision breeding
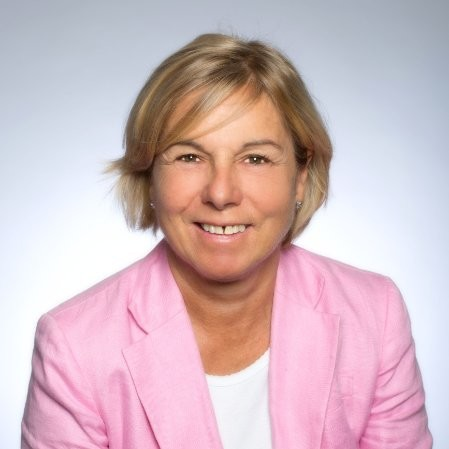
Artificial Intelligence (AI) has taken over every field of activity. Agriculture is no exception, particularly the livestock sector. Tools are already available to help identify animals, interpret and predict certain behaviours and control certain parameters.
What exactly is the current state of ‘precision breeding’? And what are its potential and development prospects?
Doctor Annick Valentin-Smith, veterinary and president and co-founder of the Vet IN Tech association, gives us the background.
Artificial Intelligence is a set of "techniques that aim to reproduce, imitate and simulate intelligence" in order to accomplish tasks or solve problems usually reserved for humans and certain animals. AI consists in particular of learning methods. Machine learning enables a machine to learn on its own "whatever the situation, without having to formally write down (or even know) all the rules"</em, thanks to a learning algorithm based on input data that will be processed to produce an output result and, "above all, to improve to produce this result".
Deep learning is one of these machine learning methods, using artificial neural networks to analyse images, sounds and videos. And it is precisely these deep learning tools that have been helping to develop so-called precision agriculture in recent years, opening up vast prospects for the challenges facing agriculture in general and livestock farming in particular.
Artificial Intelligence to meet the challenges facing agriculture
And the challenges are huge: producing enough to feed an ever-growing population, redoubling vigilance in terms of public health and meeting the challenges of climate change by reducing the environmental impact of agriculture while remaining attractive and competitive... Not forgetting respect for animal welfare, the desire of farmers to have a personal and social life, the shortage of labour...
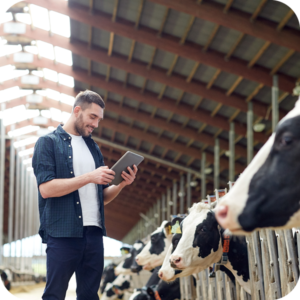
This is why agricultural players need increasingly effective and predictive tools for monitoring and production. Deep learning tools, based on the aggregation, processing and exploitation of massive data from heterogeneous sources, provide certain keys to anticipating and adapting production as effectively as possible, facilitating the work of farmers and guaranteeing and improving animal and human health.
« "Deep learning already has a strong presence in the world of livestock farming, where solutions already exist, particularly for remote monitoring, which provide real added value for farmers", explains Dr Annick Valentin-Smith, veterinary, president and co-founder of the Vet IN Tech association.
From the first warning systems...
As the vet says: ‘The arrival, from the early 2010s, of reliable and precise sensors which we have been able to fit to animals, in particular cattle - in collars or earrings among others - has made it possible to continuously capture a very large amount of data, transmit it, analyse it and exploit it.’
This initial step paved the way for the first remote monitoring, alert and decision support systems dedicated to farmers: "The first systems, which were relatively simple, were nevertheless able to spot if an animal was deviating from its usual behaviour by detecting anomalies such as fever, a lack of rumination, movement problems, etc. Above all, they enabled better monitoring of reproduction with heat detection and calving alerts", recalls Dr Valentin-Smith. The system sent a signal to the farmer indicating that a particular animal was displaying a particular behaviour. The aim: "To warn the farmer very early on, and before clinical symptoms appear, of any abnormal situation, even in a large herd, without him having to stay at the animal's bedside 24 hours a day. This is the case, for example, with ruminant thermobolus, which can detect hyperthermia in cattle, and therefore detect any respiratory problems, 3 to 5 days before clinical signs appear". The sick animal can then be isolated from the rest of the herd and treated as a preventive measure. A win-win situation for the animal, the farm and the farmer. In addition, the remote monitoring sensors can detect when an animal is in heat or giving birth, and warn the farmer: Not only is reproduction a major concern for the farmer, but it also saves him a considerable amount of time, which is all the more important given the labour shortage and recruitment difficulties" points out Annick Valentin-Smith.
... to increasingly sophisticated remote monitoring A new stage was reached a few years later, when these tools were improved by artificial intelligence, making it possible to detect "signs that are difficult for the farmer to see with the naked eye", continues Annick Valentin-Smith.
For livestock farmers, this second generation of sensors and the associated algorithms "are also an aid to health decision-making, explains Dr Valentin-Smith. As these tools are based on cases that have already occurred, they can be predictive and help with decision-making before the vet intervenes".
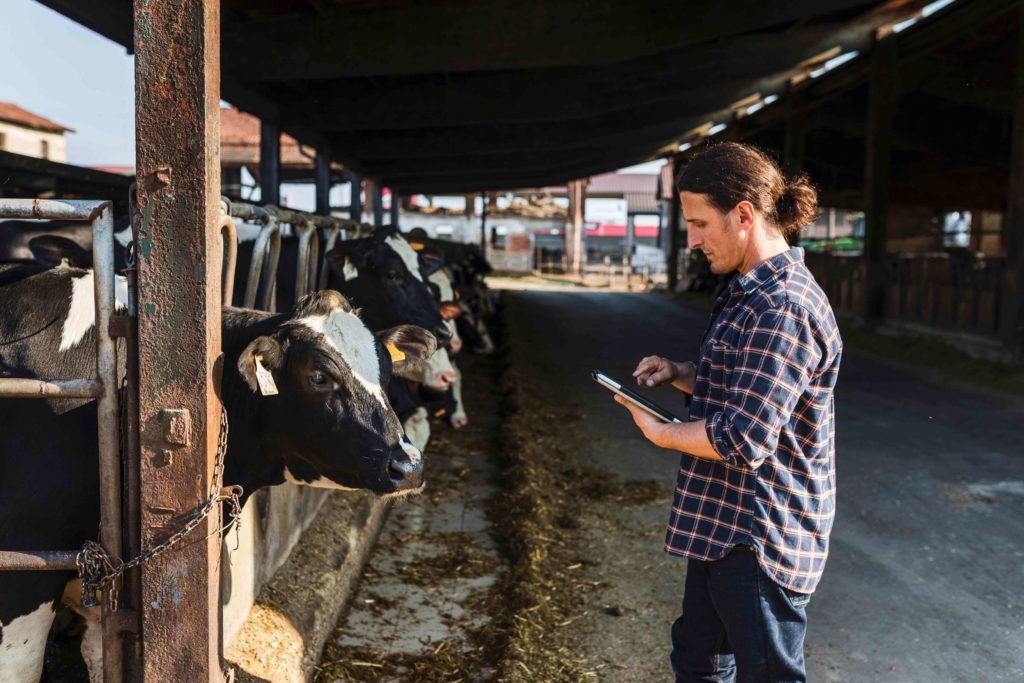
The advent of computer vision
Over the last 3 to 5 years or so, the analysis of images and videos by artificial intelligence has further extended the range of support tools available to livestock farmers: ‘Computer vision is based on AI analysis of videos produced by cameras installed in buildings, without having to equip each animal with one,’ explains the vet. These cameras - one every 100 m2 - operate on the same principle, but capture images continuously. This means we can monitor the animals‘ behaviour day and night: how they move (lameness, difficulty moving, etc.), how they lie down, prostrations, interactions, the time they spend chewing their cud, etc.’. All this enables to detect behaviour typical of certain pathologies.
Computer vision is also used to monitor cows in heat - ‘as their ovulation period is extremely short and the farmer only has a few hours in which to inseminate a cow, this offers extreme precision’.
In the purely veterinary field, Artificial Intelligence is helping to improve day-to-day practice. For example, thanks to image analysis solutions, it is now possible to automatically identify certain parasites from images obtained under a microscope.
Another potential: sound analysis
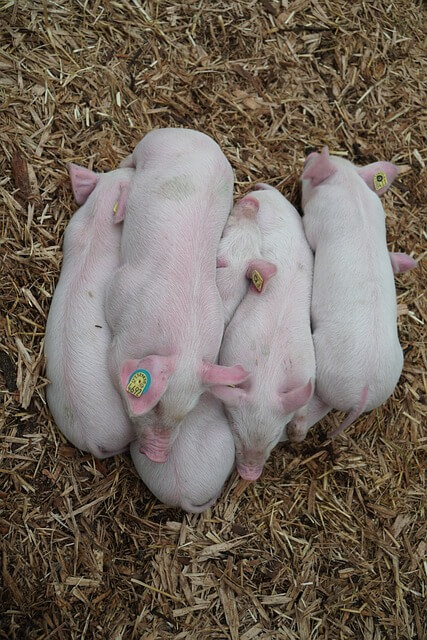
"AI sound analysis is already used in pig farms, where microphones are installed to detect aggressiveness and, above all, coughing, explains Dr Valentin-Smith. This makes it possible to spot them at a very early stage, to differentiate between them and even today to put a name to the virus or bacteria causing the cough." These systems are able to provide farmers with all the information they need, so that they can be warned well in advance of these highly contagious coughs. Translated with DeepL.com (free version)
"This is also developing on poultry farms, where AI can be used to detect anxiety, any behavioural anomalies or even intrusions," adds Annick Valentin-Smith.
Artificial Intelligence becomes more widely used in livestock farming
AI solutions dedicated to livestock farming are coming to the fore. Until now, the Consumer Electronics Show (CES) in Las Vegas, the world's largest show devoted to technological innovations, has traditionally had nothing to do with this field, but ‘at the last show, three companies, mainly South Korean, offering this type of solution were present. Mais la France n’est pas en reste avec un vivier de start-up, notamment dans l’Ouest de la France, qui ont un savoir-faire très clair en matière d’IA. Their programmers and data scientists are developing and marketing video, sound and image analysis solutions, today mainly for groups, cooperatives, breeding institutes and large-scale livestock farmers’.
It all starts with data sourcing
Whether we are talking about feeding deep learning engines to teach them to recognise images or sounds, or developing databases for generative AI, it all starts with data sourcing and quality. At this level, data intermediation platforms, such as Agdatahub for the agricultural and agri-food sectors, are a major step forward. They ensure regulatory compliance for data use authorisations at European level and securely manage data access and use. They are also an essential pivot point for the portability of datasets at national and, in future, Community level. Through the notarisation of authorisations and uses, data intermediaries play a full part in the transparency of algorithms and AI, a major challenge for developing confidence in these tools.
Promising prospects
While it's difficult to predict the future - especially when it comes to Artificial Intelligence, whose potential can be imagined to be immense - various projects are underway to optimise these deep learning tools, particularly in terms of the quality and accuracy of the cameras, which will be able, for example, to automatically measure the weight of pigs and thus warn them that they have finished fattening, where previously they would have had to be weighed. This will save time and reduce stress for the animals. ‘Generally speaking, we're moving towards cameras equipped with algorithms that will enable us to ‘see’ things more and more subtly and precisely, and so analyse increasingly detailed data,’ predicts Dr Valentin-Smith.
The aim? To warn farmers so that they can anticipate and take the most appropriate decision. ‘Ultimately, the aim is really to improve animal welfare, to treat better, to treat less, earlier and differently, for example by using far fewer antibiotics, and thus to combat antibiotic resistance more effectively.
Finally, "genetics will be greatly influenced and improved by the analysis of genes using artificial intelligence, explains Annick Valentin-Smith. The combination of AI and animal genomics can improve our understanding of the influence of genetic factors on phenotypes. The next step will be to select and ‘manufacture’ genetically improved animals on the basis of AI analyses. Supercomputers can screen all the genes of thousands of animals and will be able to make correlations between genomics and phenotypes. Today, we can already be sure that this field is going to be very, very dynamic’.
As for generative AI, on the other hand, ‘there is research going on, but it is not currently used in breeding to generate text, images, videos, etc. This does not mean that we are drawing a definitive line under it... but it's not topical at the moment! This doesn't mean that we're drawing a definitive line under it... but it's not topical at the moment! ‘concludes Annick Valentin-Smith.
The need for collaboration between all players ‘Farmers are the suppliers of the data needed for deep learning: the more the machine is fed with the farm's own data, the better the results and predictions will be. In fact, the system feeds itself, perfects itself and improves itself with this data. On the other hand, they then need to be provided with reliable, turnkey tools that are intelligent enough not to overstretch them. And that's the job of the data scientists and programmers. The solutions are also developed in conjunction with agricultural engineers and/or veterinarians in terms of their knowledge of the animals. Veterinarians are essential to the development of these new health solutions, as they are able to identify pathologies and symptoms. Veterinary surgeons will also have to learn to work with very knowledgeable breeders, even though they are more involved in curative care, since they are called in when symptoms are present. At present, not all vets are trained in preventive treatment, and this represents a major change in the mindset, training and culture of vets. All of this has to be done jointly by farmers and vets, because the different parties are totally linked and interested in the outcome through what each brings to the table. So it's vital that we work together to develop and use solutions.
NUManima, l’événement e-santé animale de Vet In Tech
Pour en savoir plus, notamment, sur les usages et l’apport de la e-santé à l’élevage, rendez-vous les 26 et 27 juin à l’École vétérinaire de Maisons-Alfort pour la 4e édition de NUManima, le congrès organisé par Vet In Tech.
Pour découvrir le programme et s’inscrire : https://vet-in-tech.com/#!numanima
- Deep learning et agriculture, étude de la Chaire AgroTIC (2018) ↩︎